-
100,000 available assets New
-
years of historical data
-
practice without risking money
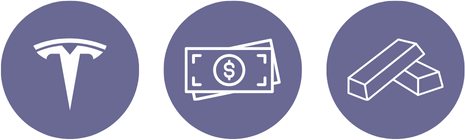
Quant Strategies & Backtesting results for QQQ
Here are some QQQ trading strategies along with their past performance. You can validate these strategies (and many more) for free on Vestinda across thousands of assets and many years of historical data.
Quant Trading Strategy: Keltner Channel and ZLEMA Trend-Following on QQQ
Based on the backtesting results, this trading strategy has shown promising performance over the period from November 2, 2016 to November 2, 2023. The strategy achieved a profit factor of 1.87, indicating that the average winning trades were 1.87 times larger than the average losing trades. With an annualized return on investment (ROI) of 10.92%, investors could expect a steady growth in their portfolio. The average holding time for trades was approximately 2 weeks and 4 days, suggesting a relatively short-term approach. The strategy had an average of 0.19 trades per week, indicating a conservative trading frequency. With 72 closed trades, the strategy had a balanced mix of both winning and losing trades, with a 50% winning trades percentage. Overall, the strategy yielded a notable return on investment of 78.02%.
Quant Trading Strategy: DMI and EMA Reversals with Confirmation on QQQ
The backtesting results statistics for the trading strategy from November 2, 2016, to November 2, 2023, indicate a profit factor of 1.02. This means that for every dollar invested, the strategy yielded a profit of $1.02. The annualized ROI stands at 0.33%, implying a modest return on investment over the entire period. On average, the strategy held positions for approximately 4 days and 9 hours. The average number of trades executed per week was 0.65, showcasing a relatively low trading frequency. With 240 closed trades in total, the strategy achieved a return on investment of 2.35%. Out of all the closed trades, 45% were profitable, suggesting room for improvement in trade selection and execution.
QQQ Backtesting: Comprehensive Step-By-Step Guide
- Obtain historical price data for QQQ, including open, high, low, and close prices.
- Select a time period for the backtest, such as one year or five years.
- Choose a specific trading strategy or indicator to backtest.
- Using the historical price data, apply the chosen strategy or indicator to generate trade signals.
- Simulate trading by executing buy and sell orders based on the generated signals.
- Calculate and analyze the performance metrics of the backtest, such as profit and loss, win rate, and drawdown.
Backtesting vs. Live QQQ Trading: A Comparison
When comparing backtested results with real-world QQQ trading, it is important to note several key factors. Backtesting is a valuable tool to assess the historical performance of a trading strategy. However, it does not guarantee future results. Real-world QQQ trading involves market dynamics and unpredictable events that cannot be accurately predicted through backtesting alone. While backtested results can provide useful insights, they can also lead to over-optimization and the assumption of unrealistic conditions. When implementing QQQ trading strategies, it is crucial to consider market liquidity, transaction costs, slippage, and other real-world factors. This analysis offers a comprehensive perspective on performance and provides a balanced assessment of QQQ trading strategies.
Unveiling QQQ Backtesting Seasonality Insights
Seasonality effects in backtesting the Invesco Qqq Trust (QQQ) have been explored to understand patterns. Over the years, researchers have noticed that certain months or seasons exhibit consistent trends. For instance, studies have revealed that the QQQ tends to perform better in the first quarter of the year compared to other quarters. This could be attributed to various factors including market sentiment, corporate earnings reports, and economic conditions. Additionally, backtesting has shown that the QQQ often experiences a "January effect," where it outperforms during the beginning of the year. However, it is important to note that these seasonality effects may not persist in the future, as market dynamics can change. Therefore, while seasonality can provide insights, it is essential to consider other factors and regularly review backtesting results to ensure accurate investment decisions.
News Events' Impact on QQQ Backtesting Results
The impact of news events on QQQ backtesting can be significant. Short sentences help convey key points. News events can cause sudden market fluctuations, influencing QQQ's performance. These events can include economic data releases, corporate earnings announcements, or geopolitical developments. Backtesting, a method to assess the historical performance of a trading strategy, allows investors to evaluate the impact of such events on QQQ's returns. By analyzing the historical data, investors can gain insights into how news events may affect QQQ's performance. However, backtesting has limitations, as it is based on past data and may not fully capture future market dynamics. Therefore, it's crucial to interpret backtested results within the context of the specific news events that occurred during the period being analyzed.
-
Create
account -
Build trading strategies
with no code -
Validate
& Backtest -
Automate
& start earning
Frequently Asked Questions
The amount of backtesting required for an ETF depends on various factors. Generally, a significant historical period of at least 5 to 10 years is needed to assess the fund's performance through different market conditions. However, the type of ETF, its investment strategy, and underlying assets may warrant even longer backtesting periods. Additionally, investors should evaluate the quality of the backtesting data, considering factors like accuracy and representativeness. Ultimately, a thorough analysis of a sufficient backtesting duration enhances confidence in an ETF's historical performance, aiding investors' decision-making process.
The best backtesting language depends on individual preferences and requirements. However, some popular options include Python, R, and MATLAB. Python is known for its simplicity, extensive libraries (such as Pandas and NumPy), and active community support. R is favored for its statistical capabilities and extensive packages like quantstrat. MATLAB is renowned for its powerful technical computing abilities and built-in functions for financial modeling. Ultimately, the choice of backtesting language should be based on personal experience, familiarity, and specific objectives.
Yes, backtesting can be conducted on QQQ margin trading platforms. These platforms typically provide historical price data for the QQQ (PowerShares QQQ Trust) exchange-traded fund, which represents the Nasdaq-100 Index. Traders can use this data to simulate their trading strategy on past market conditions and evaluate its performance. By backtesting on QQQ margin trading platforms, users can gain insights into the effectiveness of their strategies and make informed decisions about their margin trading activities.
Determining the "best" ETF chart depends on an individual's specific investment goals, risk tolerance, and market analysis. Different ETFs track various sectors, indices, or asset classes, making it crucial to select a chart that aligns with one's investing strategy. Factors to consider include historical performance, expense ratio, liquidity, and the underlying assets' composition. Conducting thorough research, seeking professional advice, and keeping a close eye on market trends and the ETF's objective will help investors make an informed decision on the best ETF chart for their needs.
Backtesting is a useful tool for evaluating trading strategies, but it may not effectively simulate black swan events in the QQQ (Invesco QQQ Trust). Black swan events refer to extremely rare and unexpected market occurrences with severe consequences. Backtesting relies on historical data to model trades and cannot account for events with no prior records. Black swan events, by their nature, are unpredictable and inherently challenging to simulate accurately. Therefore, while backtesting can provide insights into strategy performance, it may not capture the impact of black swan events on the QQQ.
Conclusion
In conclusion, QQQ backtesting is an essential tool for evaluating the performance and profitability of ETF strategies designed for QQQ. By analyzing historical data and simulating various investment techniques, investors can make informed decisions based on real-world results. However, it is important to note that backtesting is not a guarantee of future results, as real-world trading involves unpredictable events and market dynamics that cannot be accurately predicted through backtesting alone. It is crucial to consider factors such as market liquidity, transaction costs, and slippage when implementing QQQ trading strategies. Additionally, while seasonality effects and news events can provide valuable insights, it is essential to regularly review backtesting results and consider other factors for accurate investment decisions.