-
Track your
Crypto Portfolio -
Copy Crypto trading
strategies -
Build trading strategies
with no code
-
Backtest trading strategies
on Crypto, Forex, Stocks, etc. -
Demo Trading
Risk-free Paper Trading -
Automate trading strategies
with Live Trading
Automated Strategies & Backtesting results for TAN
Here are some TAN trading strategies along with their past performance. You can validate these strategies (and many more) for free on Vestinda across thousands of assets and many years of historical data.
Automated Trading Strategy: Template - LONG DEMA and Bollinger Bands on TAN
The backtesting results for the trading strategy from November 2, 2022, to November 2, 2023, reveal some interesting statistics. The profit factor stood at 0.3, indicating that the strategy generated a modest return on investment. However, the strategy experienced an annualized ROI of -21.54%, suggesting a negative performance overall. On average, positions were held for approximately 1 week and 2 days, while only 0.26 trades were executed per week. With a total of 14 closed trades, the winning trades percentage was a mere 14.29%. Nevertheless, the strategy outperformed the buy and hold approach, generating excess returns of 34.76%.
Automated Trading Strategy: VWAP and EMA Crossover or Confirmation on TAN
The backtesting results for the trading strategy from November 2, 2016, to November 2, 2023, indicate a profit factor of 1.58, highlighting the overall profitability of the strategy. The annualized ROI stands at an impressive 19.8%, showcasing the consistent returns generated over the tested period. The average holding time for trades spans 1 week and 5 days, emphasizing the short to medium-term nature of the strategy. With an average of 0.25 trades per week, the frequency of trades is relatively low. The strategy has successfully executed 93 closed trades, leading to a substantial return on investment of 141.45%. While the winning trades percentage stands at 35.48%, it outperforms the buy and hold approach by generating excess returns of 7.12%.
Algorithmic Trading: Optimizing TAN Investments
- Research and select a reliable algorithmic trading platform.
- Create an account on the platform and complete the necessary verification process.
- Connect your trading account to the algorithmic trading platform.
- Develop or find a suitable algorithmic trading strategy specifically for TAN.
- Test the trading strategy using historical data to ensure its effectiveness.
- Implement the algorithmic trading strategy on the platform for TAN.
- Monitor the performance of the algorithmic trading strategy regularly and make necessary adjustments.
Crafting an Algorithmic Trading Bot for TAN
Building a TAN algorithmic trading bot from scratch can be a challenging but rewarding endeavor. It requires a deep understanding of how the markets work and the ability to develop complex algorithms.
To start, you need to gather historical data on TAN's performance and identify patterns or trends. Next, you'll need to develop a trading strategy based on this analysis. This involves setting parameters for buying and selling TAN based on indicators like moving averages or relative strength index (RSI).
Once your strategy is in place, you can start coding the algorithm and integrating it with a trading platform that supports automated trading. Testing and backtesting are crucial to ensure the bot performs as expected and has a solid risk management system.
With a well-designed algorithmic trading bot, you can take advantage of the volatility and potential profit opportunities in the TAN market while reducing emotional biases and improving efficiency.
Market Microstructure's TAN Algorithmic Impacts
The market microstructure greatly impacts TAN algorithmic trading. High-frequency traders often exploit rapid market changes, affecting the ETF's performance. TAN's algorithmic trading relies on accurate and timely market data to execute trades efficiently. Small changes in bid-ask spreads or liquidity can significantly affect the ETF's buying and selling decisions. Additionally, the ETF is influenced by order flow imbalances, price impact, and transaction costs. In an illiquid or volatile market, TAN's algorithmic trading may experience challenges, leading to suboptimal outcomes. It is crucial for TAN algorithmic traders to monitor and adapt to changes in market microstructure to maximize the ETF's performance.
Trend-Focused Strategies for Solar ETF Trading
Trend-following approaches play a crucial role in TAN trading algorithms, which are designed to track the performance of the Invesco Solar Etf. By analyzing historical price data and market trends, these algorithms aim to identify and capitalize on upward or downward movements in the solar energy sector. Short-term trends are identified using technical indicators, such as moving averages and relative strength index (RSI), while longer-term trends are captured through the use of trendlines and support/resistance levels. These approaches enable the algorithm to adapt to changing market conditions and potentially generate higher returns by riding the momentum of the trend. However, it is important to note that trend-following strategies are not foolproof and can be susceptible to false signals or sudden reversals in the market. Therefore, risk management techniques are crucial to ensure the overall success of the TAN trading algorithms.
TAN Algorithmic Trading Primer
The TAN algorithmic trading is a strategy used specifically for Invesco Solar Etf. It leverages advanced mathematical models and automation to analyze market trends, identify potential trading opportunities, and execute trades based on predefined rules. This approach combines the advantages of technology and data analysis to make quick and objective trading decisions. The algorithm behind TAN trading continuously monitors the market, tracking various indicators such as price movements, volume, and market sentiment. By doing so, it aims to capture short-term price fluctuations and potentially generate higher returns. The main goal of algorithmic trading with TAN is to remove emotional biases and human errors from the decision-making process, allowing for more efficient and objective trading.
-
100,000 available assets New
-
years of historical data
-
practice without risking money
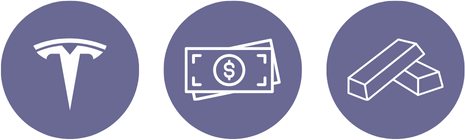
Frequently Asked Questions
Algorithmic trading, or algo trading, has the potential to be highly profitable. Its appeal lies in its ability to execute trades at high speeds with minimal human intervention. Algo trading allows for efficient execution, faster reaction to market movements, and the ability to exploit short-term opportunities. However, profitability in algo trading is not guaranteed, as it requires robust strategies, careful risk management, continuous monitoring, and adaptation to changing market conditions. Successful algo trading depends on the expertise of the trader, the quality of algorithms used, and the ability to handle potential technological glitches. When implemented effectively, algo trading can be a lucrative tool for financial institutions and individual traders.
It is possible for Algo, the cryptocurrency developed by Algorand, to reach a value of 100 dollars. However, predicting the future price of any cryptocurrency is highly speculative and depends on various factors like market demand, adoption rate, competition, and overall market conditions. While Algo has shown promising growth since its inception, it is essential to conduct comprehensive research and consider the inherent risks before making any investment decisions.
TAN algorithmic traders mitigate the impact of black swan events through various strategies. Firstly, they incorporate risk management protocols, such as stop-loss orders, to limit potential losses. Secondly, they utilize sophisticated mathematical models to analyze market trends and volatility, enabling them to make timely adjustments to their trading strategies. Additionally, they diversify their portfolios across different asset classes and geographies to reduce exposure to specific events. Lastly, they maintain constant monitoring of market conditions to swiftly adapt and respond to unforeseen circumstances. Through these measures, TAN algorithmic traders aim to minimize the impact of black swan events and protect their investments.
Slippage in algorithmic trading refers to the difference between the expected price of a trade and the actual executed price. It occurs due to market volatility, liquidity, and delays in order execution. Algorithms aim to execute trades at specific prices, but slippage can cause them to be filled at different prices, impacting profitability. Positive slippage occurs when trades are executed at better prices, while negative slippage happens when trades are filled at worse prices. Minimizing slippage is crucial for algorithmic traders, as it directly affects their trading strategy's effectiveness and overall returns.
Algorithmic trading can significantly impact liquidity in TAN markets. By using complex mathematical models and high-speed computers, algorithms can execute trades swiftly and efficiently, increasing market liquidity. The automated nature of algorithmic trading enables constant monitoring of market conditions, ensuring minimal bid-ask spreads and reducing price impact. Additionally, algorithms can identify and capitalize on trading opportunities faster than human traders, leading to more liquidity provision. Overall, algorithmic trading enhances market efficiency and liquidity in TAN markets, benefiting traders by facilitating smoother and faster execution of trades.
Some of the best programming libraries for TAN (Technically Advanced Neutral network) algorithmic trading include TensorFlow, Keras, and PyTorch. These libraries offer powerful tools for implementing and training neural networks, which are crucial in developing and optimizing trading algorithms. They provide a wide range of functionalities such as pre-built neural network architectures, automatic differentiation, and GPU acceleration, making them popular choices among algorithmic traders. Additionally, their extensive communities and comprehensive documentation further enhance their usability and support for TAN algorithmic trading.
Conclusion
In conclusion, TAN (Invesco Solar Etf) Algorithmic Trading offers investors an innovative and efficient way to capitalize on the renewable energy sector. By leveraging advanced algorithms and automation, TAN Algorithmic Trading strategies aim to exploit market inefficiencies and trends, providing potential opportunities for financial gain. However, building and implementing a successful algorithmic trading bot requires in-depth knowledge of the market, a reliable algorithmic trading platform, and thorough testing to ensure effectiveness. Additionally, monitoring and adapting to changes in the market microstructure are critical for maximizing TAN Algorithmic Trading's performance. With its ability to remove emotional biases and improve efficiency, TAN Algorithmic Trading is a promising approach for investors looking to contribute to a greener future while maximizing their returns in the solar energy sector.